Note: Woven Planet became Woven by Toyota on April 1, 2023. Note: This blog was originally published on April 7, 2022 by Level 5, which is now part of Woven Planet. This post was updated on December 13, 2022.
By Lukas Platinsky, Staff Engineer; Hugo Grimmett, Staff Product Manager; and Luca Del Pero, Engineering Manager
The latest milestones in our data-driven approach to autonomous driving
At Woven Planet, we’re using machine learning (ML) to build an autonomous driving system that improves as it observes more human driving. This is based on our Autonomy 2.0 approach, which leverages machine learning and data to solve the complex task of driving safely. This is unlike traditional systems, where engineers hand-design rules for every possible driving event.
Last year, we took a critical step in delivering on Autonomy 2.0 by using an ML model to power our motion planner, the core decision-making module of our self-driving system. We saw the ML Planner’s performance improve as we trained it on more human driving data. However, at the time we were collecting the driving data using traditional, expensive autonomous vehicle (AV) sensor suites (including lidar and radar).
Today, we are excited to share that we’ve gone two steps further. First, our ML Planner has advanced to a point where it no longer needs expensive sensors to learn from human driving; it can learn effectively from stereo camera data. Second, we developed a camera-only collection device that is 90% cheaper than the sensors we were using before. This device can be easily installed in fleets of passenger cars to gather the data we need to power autonomy development at both low cost and global scale.
Proving that camera data can power our ML Planner
We needed to prove the hypothesis that lower-fidelity camera data could be used for training our ML Planner as effectively as the high-quality data gathered by our AV sensor suite. We began by picking a fairly common scenario — stopping behind a lead vehicle — to see if we could improve its performance with low-cost data from stereo cameras. Previously, we only used data from AV sensor suites to train the ML Planner on this scenario. We mined our camera data to find examples of this scenario type, and added them to the AV data training set.
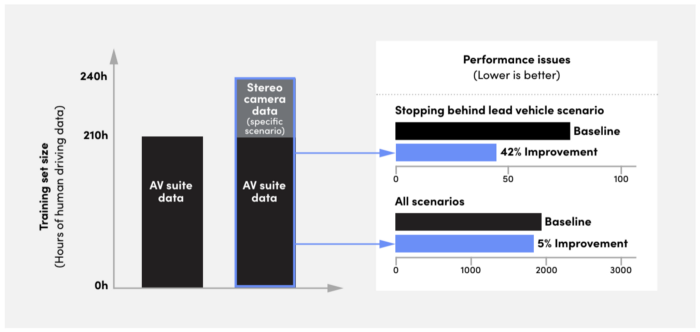
Proving our hypothesis took some work. The first time we tried using camera data, the ML Planner’s performance was 15% worse compared with training it on high-cost data only. That’s because the output of our perception algorithms was not accurate enough for the ML Planner to learn to drive from the camera data, confirming our intuition that this would be much harder to do with these lower-fidelity sensors. Our team focused on iteratively improving our perception algorithms for camera data in a tight loop with the ML Planner’s comfort and safety metrics. That allowed us to target improvements to the perception system that had the highest impact on ML Planner performance. After nine months of work, the ML Planner’s performance improved by 42% on the “stop behind lead” scenario thanks to the camera data, and by 5% across all driving scenarios. We now had hard evidence that camera data was useful for training our ML Planner.
Achieving a 5% overall performance improvement was a promising start, but the camera data was a small proportion of the total training data. Next, we wanted to know what happens if the majority of the training data comes from cameras. We increased the proportion of camera data, and witnessed a critical breakthrough: the ML Planner’s performance was comparable to when it was trained exclusively on high-cost data.
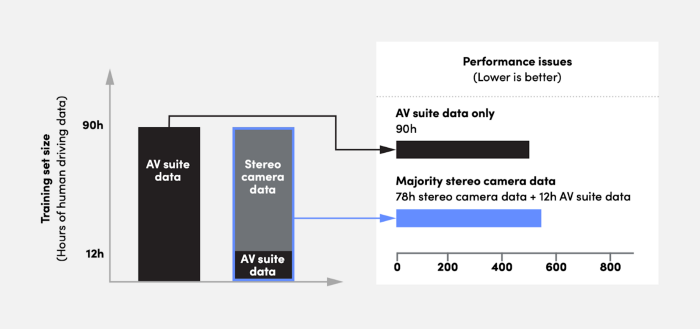
The significance of this result is profound. Not only can we add targeted amounts of camera data to improve the ML Planner on specific scenarios, we can also train the ML Planner effectively even when camera data is our primary source.
We are now confident that our ML-based approach can learn from low-cost cameras installed on human-driven cars. Alongside this, we began scaling up our ability to collect camera-based data.
Meet the device collecting data at scale
To begin collecting data at scale, we built our own in-house device equipped with stereo cameras. The cameras are housed in a roof pod that makes it easy to non-invasively mount them on fleets of human-driven passenger vehicles. This allows us to deploy them at scale — we’ll be rolling them out in the Bay Area and beyond in 2022.
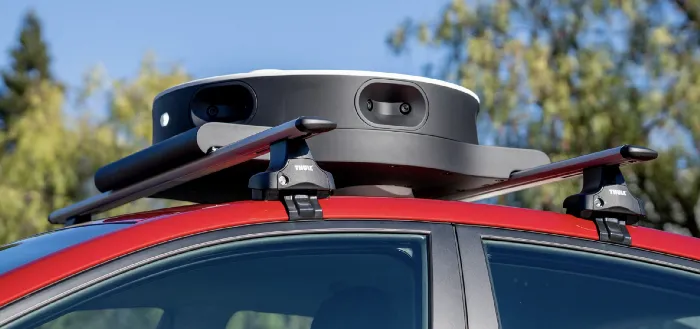
Our low-cost sensor suite is made up of only stereo cameras. These vehicles were selected prior to our joining Woven Planet, and we look forward to transitioning the devices to a fleet of Toyota vehicles shortly.
Accelerating forward with a scalable approach to AV development
By design, we can train our ML Planner on a combination of data collected from different sensor configurations with varying levels of fidelity.
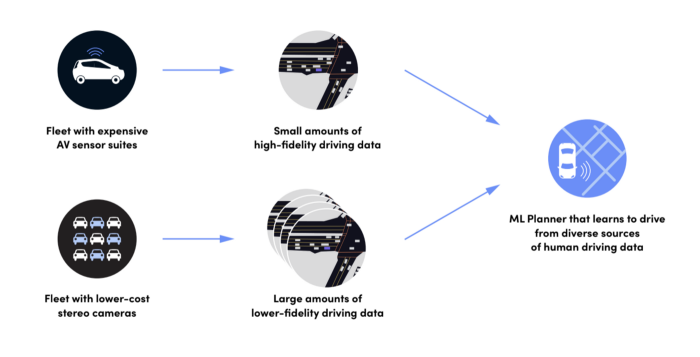
So far, this allowed us to use a mix of data from both AV sensors and camera sensors. It also gives us the opportunity to leverage Woven Planet’s unique advantage: access to diverse driving data at unprecedented scale through Toyota, one of the largest automakers in the world. Access to this sheer amount of human driving data will enable us to bring our ML-first Autonomy 2.0 approach to production.
Join us!
We’re looking for talented engineers to help us on this journey. If you’d like to join us, we’re hiring!